Time Series Solution Manual
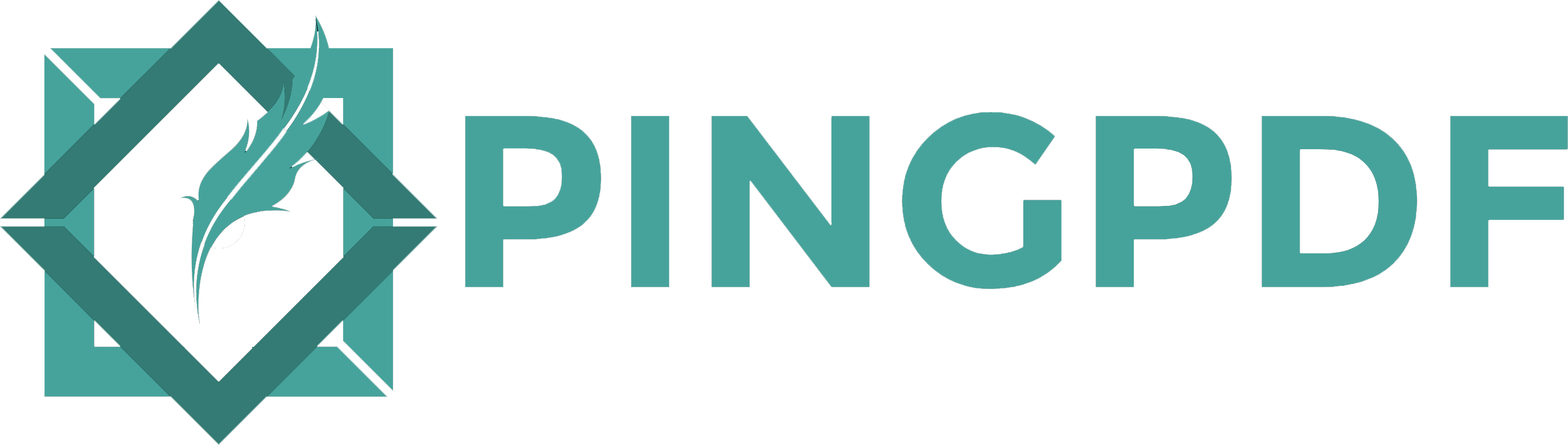
This page is updated frequently at any time and contains information about books, past and current users, and software. This page also contains links to all known errors in the book, accompanying slides, and software. Because the manual solution is distributed electronically, all known errors are promptly corrected and no error lists are maintained. Instructors are encouraged to visit this site regularly; they may also register on this site to be notified of important changes by email. (read: privacy policy).
Library Archives of.
An accessible introduction to the most current thinking in and practicality of forecasting techniques in the context of time-oriented data Analyzing time-oriented data and forecasting are among the most important problems that analysts face across many fields, ranging from finance and economics to production operations and the natural sciences. As a result, there is a widespread need for large groups of people in a variety of fields to understand the basic concepts of time series analysis and forecasting. Introduction to Time Series Analysis and Forecasting presents the time series analysis branch of applied statistics as the underlying methodology for developing practical forecasts, and it also bridges the gap between theory and practice by equipping readers with the tools needed to analyze time-oriented data and construct useful, short- to medium-term, statistically based forecasts. Preface ix 1.
Introduction to Forecasting 1 1.1 The Nature and Uses of Forecasts, 1 1.2 Some Examples of Time Series, 5 1.3 The Forecasting Process, 12 1.4 Resources for Forecasting, 14 2. Statistics Background for Forecasting 18 2.1 Introduction, 18 2.2 Graphical Displays, 19 2.3 Numerical Description of Time Series Data, 25 2.4 Use of Data Transformations and Adjustments, 34 2.5 General Approach to Time Series Modeling and Forecasting,46 2.6 Evaluating and Monitoring Forecasting Model Performance,49 3.
Time Series Brockwell Solution Manual
Regression Analysis and Forecasting 73 3.1 Introduction, 73 3.2 Least Squares Estimation in Linear Regression Models, 75 3.3 Statistical Inference in Linear Regression, 84 3.4 Prediction of New Observations, 96 3.5 Model Adequacy Checking, 98 3.6 Variable Selection Methods in Regression, 106 3.7 Generalized and Weighted Least Squares, 111 3.8 Regression Models for General Time Series Data, 133 4. Exponential Smoothing Methods 171 4.1 Introduction, 171 4.2 First-Order Exponential Smoothing, 176 4.3 Modeling Time Series Data, 180 4.4 Second-Order Exponential Smoothing, 183 4.5 Higher-Order Exponential Smoothing, 193 4.6 Forecasting, 193 4.7 Exponential Smoothing for Seasonal Data, 210 4.8 Exponential Smoothers and ARIMA Models, 217 5. Autoregressive Integrated Moving Average (ARIMA) Models231 5.1 Introduction, 231 5.2 Linear Models for Stationary Time Series, 231 5.3 Finite Order Moving Average (MA) Processes, 235 5.4 Finite Order Autoregressive Processes, 239 5.5 Mixed Autoregressive-Moving Average (ARMA) Processes,253 5.6 Nonstationary Processes, 256 5.7 Time Series Model Building, 265 5.8 Forecasting ARIMA Processes, 275 5.9 Seasonal Processes, 282 5.10 Final Comments, 286 6. Transfer Functions and Intervention Models 299 6.1 Introduction, 299 6.2 Transfer Function Models, 300 6.3 Transfer Function-Noise Models, 307 6.4 Cross Correlation Function, 307 6.5 Model Specification, 309 6.6 Forecasting with Transfer Function-Noise Models,322 6.7 Intervention Analysis, 330 7. Survey of Other Forecasting Methods 343 7.1 Multivariate Time Series Models and Forecasting, 343 7.2 State Space Models, 350 7.3 ARCH and GARCH Models, 355 7.4 Direct Forecasting of Percentiles, 359 7.5 Combining Forecasts to Improve Prediction Performance,365 7.6 Aggregation and Disaggregation of Forecasts, 369 7.7 Neural Networks and Forecasting, 372 7.8 Some Comments on Practical Implementation and Use ofStatistical Forecasting Procedures, 375 Appendix A. Statistical Tables 387 Appendix B.
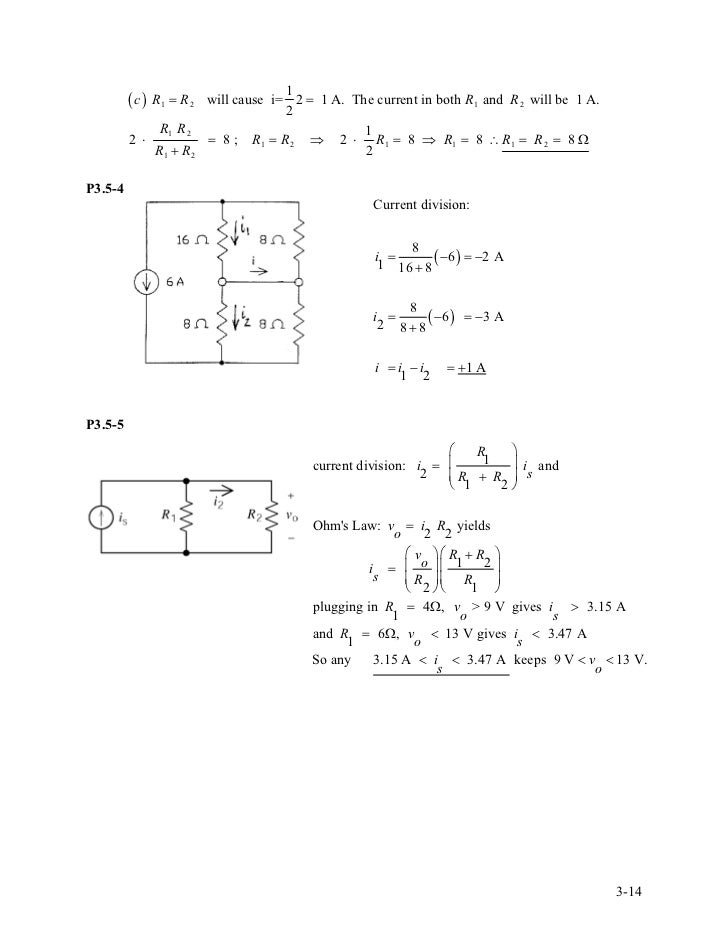
Time Series Shumway Solution Manual
Data Sets for Exercises 407 Bibliography 437 Index 443. Montgomery, PhD, is Regents' Professor of Industrial Engineering and Statistics at Arizona State University.
Calculus early transcendentals briggs solution manual. Montgomery has over thirty years of academic and consulting experience and has devoted his research to engineering statistics, specifically the design and analysis of experiments, statistical methods for process monitoring and optimization, and the analysis of time-oriented data. He has authored or coauthored over 190 journal articles and eleven books, including Introduction to Linear Regression Analysis, Fourth Edition and Generalized Linear Models: With Applications in Engineering and the Sciences, both published by Wiley. Jennings, PhD, is a Process Design Consultant with Bank of America.
An active member of both the American Statistical Association and the American Society for Quality, her areas of research and professional interest include Six Sigma; modeling and analysis; and process control and improvement. Jennings earned her PhD in industrial engineering from Arizona State University. Murat Kulahci, PhD, is Associate Professor in Informatics and Mathematical Modelling at the Technical University of Denmark. He has authored or coauthored over thirty journal articles in the areas of time series analysis, design of experiments, and statistical process control and monitoring. Montgomery, Georgia Institute of Technology; C. Jennings, Bank of America, USA; M. Kulahci, Technical University of Denmark.
Comments are closed.